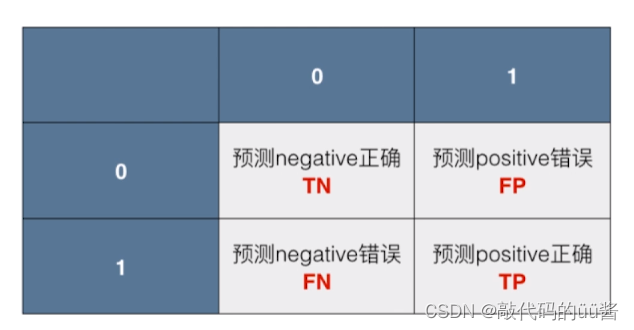
机器学习评估分类结果——精准率、召回率、F1Score
机器学习评估分类结果——混淆矩阵、精准率、召回率、F1Score
目录
1、分类准确度问题
假设有一个癌症预测系统,输入体检信息,可以判断是否有癌症。如果癌症产生的概率只有0.1%,那么系统预测所有人都是健康,即可达到99.9%的准确率。但显然这样的机器学习系统是失败的,因为对于极度偏斜(Skewed Data)的数据,只使用分类准确度是远远不够的。
针对这样的问题,我们需要引入其他指标来评估机器学习分类结果的好坏。
2、混淆矩阵
对于二分类问题,混淆矩阵是2x2,分别是0和1,其中每一行代表真实值,每一列代表预测值。
假设现在有10000个人的信息,预测他们患癌症的概率。混淆矩阵如下所示,其中0代表不患病,1代表患病。对于这10000个人中,有9978人没患病,其中有12个人也没患病,但是算法错误的将它们预测成了患病。同时,有2个人患病了但是算法错误的预测成了没患病,最后有8个人真实患病,算法也正确识别出。
3、精准率、召回率及实现
精准率precision = ,即预测数据中预测正确的数量就是精准率。
召回率recall = ,即真实发生数据预测正确的数量就是召回率。
代码示例:
import numpy as np
from sklearn import datasets
digits = datasets.load_digits()
X = digits.data
y = digits.target.copy()
#通过该方式具有了一个极度偏斜的数据
y[digits.target==9] = 1
y[digits.target!=9] = 0
from sklearn.model_selection import train_test_split
X_train, X_test, y_train, y_test = train_test_split(X, y, random_state=666)
from sklearn.linear_model import LogisticRegression
log_reg = LogisticRegression()
log_reg.fit(X_train, y_train)
log_reg.score(X_test, y_test)
y_log_predict = log_reg.predict(X_test)
def TN(y_true, y_predict):
assert len(y_true) == len(y_predict)
return np.sum((y_true == 0) & (y_predict == 0))
def FP(y_true, y_predict):
assert len(y_true) == len(y_predict)
return np.sum((y_true == 0) & (y_predict == 1))
def FN(y_true, y_predict):
assert len(y_true) == len(y_predict)
return np.sum((y_true == 1) & (y_predict == 0))
def TP(y_true, y_predict):
assert len(y_true) == len(y_predict)
return np.sum((y_true == 1) & (y_predict == 1))
#混淆矩阵
def confusion_matrix(y_true, y_predict):
return np.array([
[TN(y_true, y_predict), FP(y_true, y_predict)],
[FN(y_true, y_predict), TP(y_true, y_predict)]
])
confusion_matrix(y_test, y_log_predict)
#精准率
def precision_score(y_true, y_predict):
tp = TP(y_true, y_predict)
fp = FP(y_true, y_predict)
try:
return tp / (tp + fp)
except:
return 0.0
precision_score(y_test, y_log_predict)
#召回率
def recall_score(y_true, y_predict):
tp = TP(y_true, y_predict)
fn = FN(y_true, y_predict)
try:
return tp / (tp + fn)
except:
return 0.0
recall_score(y_test, y_log_predict)
#sklearn中实现混淆矩阵、精准率、召回率
'''
from sklearn.metrics import confusion_matrix
confusion_matrix(y_test, y_log_predict)
from sklearn.metrics import precision_score
precision_score(y_test, y_log_predict)
from sklearn.metrics import recall_score
recall_score(y_test, y_log_predict)
'''
运行结果:
4、F1Score
F1Score指标就是综合精准率和召回率两个指标,计算方法为F1 = ,它是precision和recall的调和平均值。
代码示例:
import numpy as np
def f1_score(precision, recall):
try:
return 2 * precision * recall / (precision + recall)
except:
return 0.0
f1 = f1_score(ps, rs) #ps精准率,rs召回率
#sklearn中实现
'''
from sklearn.metrics import f1_score
f1_score(y_test, y_log_predict)
'''
5、精准率-召回率曲线
代码示例:
#决策分数值decision_function
decision_scores = log_reg.decision_function(X_test)
precisions = [] #存储精准率
recalls = [] #存储召回率
#横纵坐标不同域值
thresholds = np.arange(np.min(decision_scores), np.max(decision_scores), 0.1)
for threshold in thresholds:
y_predict = np.array(decision_scores >= threshold, dtype='int')
precisions.append(precision_score(y_test, y_predict))
recalls.append(recall_score(y_test, y_predict))
import matplotlib.pyplot as plt
#绘制Precision-Recall曲线
plt.plot(precisions, recalls)
plt.show()
#scikit-learn中的Precision-Recall曲线
'''
from sklearn.metrics import precision_recall_curve
precisions, recalls, thresholds = precision_recall_curve(y_test, decision_scores)
plt.plot(thresholds, precisions[:-1])
plt.plot(thresholds, recalls[:-1])
plt.show()
'''
运行结果:
可以看到,随着精准率的增加,召回率是逐渐降低的,说明精准率和召回率是互相牵制的两个指标。同时也可知该曲线急剧下降的点是它们平衡的最好位置。
6、ROC曲线
首先引入两个指标,误报率(FPR)和召回率(TPR)。其中FPR = ,它是指是被错误地预测为正类的样本占所有实际为负类的样本的比例。TPR =
,它是指被正确地预测为正类的样本占所有实际为正类的样本的比例。
代码示例:
from math import sqrt
def TPR(y_true, y_predict):
tp = TP(y_true, y_predict)
fn = FN(y_true, y_predict)
try:
return tp / (tp + fn)
except:
return 0.
fprs = []
tprs = []
thresholds = np.arange(np.min(decision_scores), np.max(decision_scores), 0.1)
for threshold in thresholds:
y_predict = np.array(decision_scores >= threshold, dtype='int')
fprs.append(FPR(y_test, y_predict))
tprs.append(TPR(y_test, y_predict))
#生产ROC曲线
plt.plot(fprs, tprs)
plt.show()
#scikit-learn中的ROC
'''
from sklearn.metrics import roc_curve
fprs, tprs, thresholds = roc_curve(y_test, decision_scores)
plt.plot(fprs, tprs)
plt.show()
#曲线下方面积
from sklearn.metrics import roc_auc_score
roc_auc_score(y_test, decision_scores)
'''
运行结果:
可以看出,随着FPR的增加,TPR的值也在随着增高。对于ROC曲线,曲线下面的面积越大,说明模型的分类效果越好。因此,当我们比较两个模型孰优孰劣时,只需要选择曲线下方面积最大的那个模型。
更多推荐
所有评论(0)