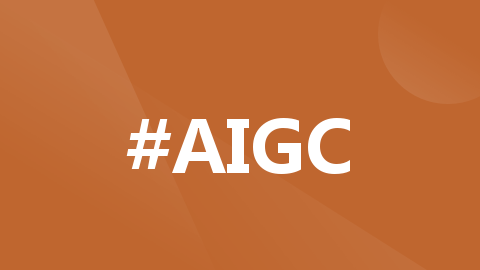
【AIGC】因果注意力(Causal Attention)原理及其代码实现
因果注意力(Causal Attention)是一种自注意力机制,广泛应用于自回归模型中,尤其是在自然语言处理和时间序列预测等任务中。它的核心思想是在生成每个时间步的输出时,只关注当前时间步及之前的时间步,确保生成过程的因果性,从而避免模型在预测时依赖未来的信息。
·
概述
因果注意力(Causal Attention)是一种自注意力机制,广泛应用于自回归模型中,尤其是在自然语言处理和时间序列预测等任务中。它的核心思想是在生成每个时间步的输出时,只关注当前时间步及之前的时间步,确保生成过程的因果性,从而避免模型在预测时依赖未来的信息。
工作原理
因果注意力的工作原理是通过掩码矩阵限制模型在计算每个时间步的注意力时,只关注当前时间步及之前的内容。具体地,掩码矩阵是一个下三角矩阵,其上三角部分为0,其余部分为1。这样,在计算注意力分布时,掩码矩阵将未来时间步的注意力得分设置为非常大的负值(-inf
),使得这些位置在 softmax
操作后接近于零,从而不会对最终的输出产生影响。
掩码矩阵示例
掩码矩阵的结构如下:
[
[1, 0, 0, 0],
[1, 1, 0, 0],
[1, 1, 1, 0],
[1, 1, 1, 1]
]
该掩码矩阵确保每个时间步仅关注当前时间步及之前的时间步,维持因果性。
NumPy实现
以下是基于NumPy的因果注意力机制实现代码:
import numpy as np
def softmax(x):
"""Compute the softmax of vector x in a numerically stable way."""
shift_x = x - np.max(x, axis=-1, keepdims=True)
exp_x = np.exp(shift_x)
softmax_x = exp_x / np.sum(exp_x, axis=-1, keepdims=True)
return softmax_x
def causal_self_attention(Q, K, V, mask):
"""
计算因果自注意力
:param Q: 查询矩阵
:param K: 键矩阵
:param V: 值矩阵
:param mask: 因果掩码矩阵,上三角为0,其余为1
:return: 自注意力的输出
"""
dim_key = K.shape[-1]
# 计算未掩码的注意力得分
attention_scores = np.matmul(Q, K.transpose(0, 2, 1)) / (np.sqrt(dim_key) + 1e-9)
# 应用因果掩码,将mask为0的位置设置为非常大的负值
attention_scores = np.where(mask == 0, -np.inf, attention_scores)
# 使用数值稳定的softmax
attention_weights = softmax(attention_scores)
# 确保无效值处理后不会影响计算结果
attention_weights = np.nan_to_num(attention_weights, nan=0.0, posinf=0.0, neginf=0.0)
# 加权求和得到输出
output = np.matmul(attention_weights, V)
return output
# 示例用法
batch_size = 2
seq_length = 4
dim = 8
Q = np.random.rand(batch_size, seq_length, dim)
K = np.random.rand(batch_size, seq_length, dim)
V = np.random.rand(batch_size, seq_length, dim)
# 创建一个上三角掩码矩阵
mask = np.triu(np.ones((seq_length, seq_length)), k=1)[np.newaxis, np.newaxis, :, :]
# 调用causal_self_attention函数
output = causal_self_attention(Q, K, V, mask)
print(output)
关键点
- 掩码矩阵:通过上三角掩码矩阵实现因果性,确保模型在生成每个时间步时只能关注当前及之前的时间步。
- 数值稳定性:在
softmax
计算中,通过减去最大值来提高数值稳定性,避免溢出问题。 - 无效值处理:在计算注意力权重时,使用
np.nan_to_num
处理无效值,确保结果的有效性。
应用场景
- 自回归语言模型:如GPT系列,在生成下一个词时,只能依赖已生成的词。
- 语音生成:如WaveNet,在生成下一帧语音数据时,只能依赖之前的帧。
- 时间序列预测:在预测过程中,不依赖未来时间步,确保预测的因果性。
Code
代码已上传至:AI_With_NumPy
此项目汇集了更多AI相关的算法实现,供大家学习参考使用,欢迎点赞收藏👏🏻
备注
个人水平有限,有问题随时交流~
更多推荐
所有评论(0)