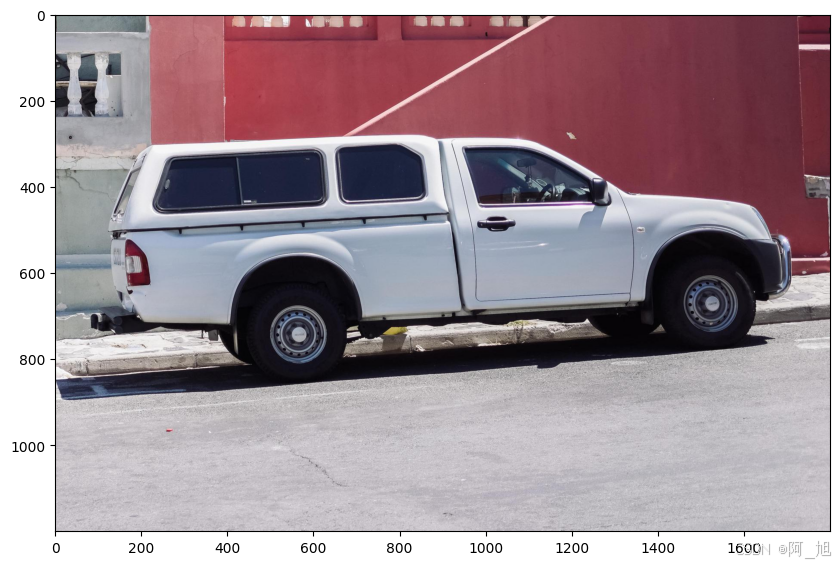
SAM2(segment anything 2)使用指南【1】:使用SAM2分割图片,根据不同提示信息分割图片
SAM2(segment anything 2)使用指南【1】:使用SAM2分割图片,根据不同提示信息分割图片
《------往期经典推荐------》
二、机器学习实战专栏【链接】,已更新31期,欢迎关注,持续更新中~~
三、深度学习【Pytorch】专栏【链接】
四、【Stable Diffusion绘画系列】专栏【链接】
五、YOLOv8改进专栏【链接】,持续更新中~~
六、YOLO性能对比专栏【链接】,持续更新中~
《------正文------》
目录
引言
本文主要介绍如何使用最新的SAM2分割大模型进行图片分割
。分割图片的功能与之前的SAM基本一致。后续会继续介绍使用SAM2对视频进行分割。
关于原始SAM的使用和介绍可以参考我之前的文章。
1.《【CV大模型SAM(Segment-Anything)】真是太强大了,分割一切的SAM大模型使用方法:可通过不同的提示得到想要的分割目标》
SAM2简介
最新的SAM2分割大模型(Segment Anything Model 2)
是由Meta开发的一个先进的图像和视频分割模型
。相比于第一代SAM模型,SAM2在多个方面实现了显著的改进:
- 支持视频分割:SAM2的一个重要进展是它的能力从图像分割扩展到了视频分割。这意味着它能够处理视频中的对象,而不仅仅是静态图像。
- 实时处理任意长视频:SAM2能够实时处理任意长度的视频,这在实际应用中非常有用,尤其是在需要快速响应的场景中。
- Zero-shot泛化:即使是在视频中没有见过的对象,SAM2也能实现有效的分割和追踪,这显示了其强大的泛化能力。
- 分割和追踪准确性提升:与第一代模型相比,SAM2在分割和追踪准确性方面有了显著提升。
解决遮挡问题:在视频分割中,对象可能会被遮挡,SAM2能够有效地处理这种情况,即使在物体暂时遮挡的情况下也能帮助分割物体。 - 交互式分割过程:SAM2的分割过程是交互式的,用户可以通过点击来选择和细化目标对象,模型会根据这些提示自动将分割传播到视频的后续帧。
- 引入记忆模块:为了处理视频中的对象,SAM2引入了流式记忆模块,这使得模型能够利用先前帧的信息来辅助当前帧的分割任务。
- 数据集和模型的开源:Meta此次开源的数据集包含51000个真实世界视频和600000个时空掩码,这是迄今为止同类数据集中规模最大的。同时,模型代码、权重和数据集均遵循Apache 2.0许可协议开源。
总的来说,SAM2的这些改进使其成为一个更加强大和灵活的工具,适用于广泛的图像和视频分割任务
环境配置
源码地址:https://github.com/facebookresearch/segment-anything-2
pip install -e .
或者
pip install --no-build-isolation -e .
环境配置中会遇到各种奇葩问题,解决办法可以参考之前写的博客《SAM2环境配置问题汇总》。希望能帮助各位小伙伴顺利运行。
使用SAM2根据不同提示信息分割图片
Segment Anything Model 2 (SAM 2) 根据指示所需对象的提示预测对象掩码。
该模型首先将图像转换为图像嵌入,从而允许根据提示有效地生成高质量的蒙版。
SAM2ImagePredictor 类为模型提供了一个简单的接口,用于提示模型。它允许用户首先使用 set_image 方法设置图像,该方法计算必要的图像嵌入。然后,可以通过 predict 方法提供提示,以根据这些提示有效地预测掩码。该模型可以将点提示和框提示以及上一次预测迭代的掩码作为输入。
初始化设置
相关库导入以及定义辅助函数
import torch
import numpy as np
import matplotlib.pyplot as plt
from PIL import Image
from sam2.build_sam import build_sam2
from sam2.sam2_image_predictor import SAM2ImagePredictor
# use bfloat16 for the entire notebook
torch.autocast(device_type="cuda", dtype=torch.bfloat16).__enter__()
if torch.cuda.get_device_properties(0).major >= 8:
# turn on tfloat32 for Ampere GPUs (https://pytorch.org/docs/stable/notes/cuda.html#tensorfloat-32-tf32-on-ampere-devices)
torch.backends.cuda.matmul.allow_tf32 = True
torch.backends.cudnn.allow_tf32 = True
def show_mask(mask, ax, random_color=False, borders = True):
# 显示遮罩
if random_color:
color = np.concatenate([np.random.random(3), np.array([0.6])], axis=0)
else:
color = np.array([30/255, 144/255, 255/255, 0.6])
h, w = mask.shape[-2:]
mask = mask.astype(np.uint8)
mask_image = mask.reshape(h, w, 1) * color.reshape(1, 1, -1)
if borders:
import cv2
contours, _ = cv2.findContours(mask,cv2.RETR_EXTERNAL, cv2.CHAIN_APPROX_NONE)
# Try to smooth contours
contours = [cv2.approxPolyDP(contour, epsilon=0.01, closed=True) for contour in contours]
mask_image = cv2.drawContours(mask_image, contours, -1, (1, 1, 1, 0.5), thickness=2)
ax.imshow(mask_image)
def show_points(coords, labels, ax, marker_size=375):
# 显示提示点:前景点为绿色,背景为红色
pos_points = coords[labels==1]
neg_points = coords[labels==0]
ax.scatter(pos_points[:, 0], pos_points[:, 1], color='green', marker='*', s=marker_size, edgecolor='white', linewidth=1.25)
ax.scatter(neg_points[:, 0], neg_points[:, 1], color='red', marker='*', s=marker_size, edgecolor='white', linewidth=1.25)
def show_box(box, ax):
# 显示坐标框
x0, y0 = box[0], box[1]
w, h = box[2] - box[0], box[3] - box[1]
ax.add_patch(plt.Rectangle((x0, y0), w, h, edgecolor='green', facecolor=(0, 0, 0, 0), lw=2))
def show_masks(image, masks, scores, point_coords=None, box_coords=None, input_labels=None, borders=True):
for i, (mask, score) in enumerate(zip(masks, scores)):
plt.figure(figsize=(10, 10))
plt.imshow(image)
show_mask(mask, plt.gca(), borders=borders)
if point_coords is not None:
assert input_labels is not None
show_points(point_coords, input_labels, plt.gca())
if box_coords is not None:
# boxes
show_box(box_coords, plt.gca())
if len(scores) > 1:
plt.title(f"Mask {i+1}, Score: {score:.3f}", fontsize=18)
plt.axis('off')
plt.show()
读取图片
image = Image.open('images/truck.jpg')
image = np.array(image.convert("RGB"))
plt.figure(figsize=(10, 10))
plt.imshow(image)
plt.axis('on')
plt.show()
初始化SAM2模型
首先,加载 SAM 2 模型和预测器。注意更改sam2_checkpoint的模型路径或名称。建议在 CUDA 上运行并使用默认模型以获得最佳结果。
# SAM2模型和配置文件
sam2_checkpoint = "checkpoints/sam2_hiera_tiny.pt"
model_cfg = "sam2_hiera_t.yaml"
sam2_model = build_sam2(model_cfg, sam2_checkpoint, device="cuda")
predictor = SAM2ImagePredictor(sam2_model)
通过调用 SAM2ImagePredictor.set_image 处理图像以生成图像嵌入。SAM2ImagePredictor 会记住此嵌入,并将其用于后续掩码预测。
predictor.set_image(image)
方法一:单点提示进行预测
假如我们要选择卡车,首先在其上选择一个点。点以 (x,y) 格式输入到模型中,并带有标签 1(前景点)或 0(背景点)。
可输入多个点;这里我们只使用一个。所选点将在图像上显示为星号。
input_point = np.array([[500, 375]])
input_label = np.array([1])
plt.figure(figsize=(10, 10))
plt.imshow(image)
show_points(input_point, input_label, plt.gca())
plt.axis('on')
plt.show()
使用 SAM2ImagePredictor.predict 进行预测。该模型返回掩码masks、这些掩码的预测分数scores以及可传递给下一次预测迭代的低分辨率掩码日志logits。
masks, scores, logits = predictor.predict(
point_coords=input_point,
point_labels=input_label,
multimask_output=True,
)
sorted_ind = np.argsort(scores)[::-1]
masks = masks[sorted_ind]
scores = scores[sorted_ind]
logits = logits[sorted_ind]
使用 multimask_output=True(默认设置
),SAM 2 输出 3 个掩码,其中分数给出了模型自己对这些掩码质量的估计。此设置适用于不明确的输入提示,并帮助模型消除与提示一致的不同对象的歧义。如果为 False,它将返回一个掩码。对于模棱两可的提示,例如单个点,即使只需要单个掩码,也建议使用 multimask_output=True;可以通过选择分数最高的一个来选择最佳的单一掩模。这通常会带来更好的遮罩。
masks.shape # (number_of_masks) x H x W
(3, 1200, 1800)
# 显示分割结果
show_masks(image, masks, scores, point_coords=input_point, input_labels=input_label, borders=True)
方法二:多提示点分割
单个输入点是不明确的,并且模型返回了与其一致的多个对象。为了获得单个对象,可以提供多个点。
当指定具有多个提示的单个对象时,可以通过设置 multimask_output=False 来获取单个掩码
。
# 设置两个正向提示点
input_point = np.array([[500, 375], [1125, 625]])
input_label = np.array([1, 1])
mask_input = logits[np.argmax(scores), :, :] # Choose the model's best mask
masks, scores, _ = predictor.predict(
point_coords=input_point,
point_labels=input_label,
mask_input=mask_input[None, :, :],
multimask_output=False,
)
masks.shape
(1, 1200, 1800)
show_masks(image, masks, scores, point_coords=input_point, input_labels=input_label)
如果要排除汽车并仅指定窗户,可以提供一个背景点(标签为 0,此处显示为红色)。
# 设置1个正向提示点和1个负向提示点
input_point = np.array([[500, 375], [1125, 625]])
input_label = np.array([1, 0])
mask_input = logits[np.argmax(scores), :, :] # Choose the model's best mask
masks, scores, _ = predictor.predict(
point_coords=input_point,
point_labels=input_label,
mask_input=mask_input[None, :, :],
multimask_output=False,
)
show_masks(image, masks, scores, point_coords=input_point, input_labels=input_label)
方法三:用框指定一个特定分割对象
可以使用单个提示框进行输入,用于分割。
# 轮胎的提示框
input_box = np.array([425, 600, 700, 875])
masks, scores, _ = predictor.predict(
point_coords=None,
point_labels=None,
box=input_box[None, :],
multimask_output=False,
)
show_masks(image, masks, scores, box_coords=input_box)
方法四:点与框结合进行分割
点和框可以组合在一起,只需将两种类型的提示都包含到预测器中即可。在这里,这可以用来只选择卡车的轮胎,而不是整个车轮。
# 剔除轮胎中心区域
# 轮胎框
input_box = np.array([425, 600, 700, 875])
# 中心区域的负向提示点
input_point = np.array([[575, 750]])
input_label = np.array([0])
masks, scores, logits = predictor.predict(
point_coords=input_point,
point_labels=input_label,
box=input_box,
multimask_output=False,
)
show_masks(image, masks, scores, box_coords=input_box, point_coords=input_point, input_labels=input_label)
方法五:多提示框信息输入
SAM2ImagePredictor 可以使用 predict 方法对同一图像接收多个输入提示。例如,想象一下,我们有一个来自对象检测器的多个框输出。
input_boxes = np.array([
[75, 275, 1725, 850],
[425, 600, 700, 875],
[1375, 550, 1650, 800],
[1240, 675, 1400, 750],
])
masks, scores, _ = predictor.predict(
point_coords=None,
point_labels=None,
box=input_boxes,
multimask_output=False,
)
masks.shape # (batch_size) x (num_predicted_masks_per_input) x H x W
(4, 1, 1200, 1800)
plt.figure(figsize=(10, 10))
plt.imshow(image)
for mask in masks:
show_mask(mask.squeeze(0), plt.gca(), random_color=True)
for box in input_boxes:
show_box(box, plt.gca())
plt.axis('off')
plt.show()
图片批量分割推理
如果所有提示都提前可用,则可以直接以端到端方式运行 SAM 2。这也允许对图像进行批处理。
# 图片1
image1 = image # truck.jpg from above
image1_boxes = np.array([
[75, 275, 1725, 850],
[425, 600, 700, 875],
[1375, 550, 1650, 800],
[1240, 675, 1400, 750],
])
#图片2
image2 = Image.open('images/groceries.jpg')
image2 = np.array(image2.convert("RGB"))
image2_boxes = np.array([
[450, 170, 520, 350],
[350, 190, 450, 350],
[500, 170, 580, 350],
[580, 170, 640, 350],
])
#图片与提示信息列表
img_batch = [image1, image2]
boxes_batch = [image1_boxes, image2_boxes]
predictor.set_image_batch(img_batch)
masks_batch, scores_batch, _ = predictor.predict_batch(
None,
None,
box_batch=boxes_batch,
multimask_output=False
)
for image, boxes, masks in zip(img_batch, boxes_batch, masks_batch):
plt.figure(figsize=(10, 10))
plt.imshow(image)
for mask in masks:
show_mask(mask.squeeze(0), plt.gca(), random_color=True)
for box in boxes:
show_box(box, plt.gca())
同样,我们可以在一批图像上定义一批点提示
image1 = image # truck.jpg from above
image1_pts = np.array([
[[500, 375]],
[[650, 750]]
]) # Bx1x2 where B corresponds to number of objects
image1_labels = np.array([[1], [1]])
image2_pts = np.array([
[[400, 300]],
[[630, 300]],
])
image2_labels = np.array([[1], [1]])
pts_batch = [image1_pts, image2_pts]
labels_batch = [image1_labels, image2_labels]
masks_batch, scores_batch, _ = predictor.predict_batch(pts_batch, labels_batch, box_batch=None, multimask_output=True)
# Select the best single mask per object
best_masks = []
for masks, scores in zip(masks_batch,scores_batch):
best_masks.append(masks[range(len(masks)), np.argmax(scores, axis=-1)])
for image, points, labels, masks in zip(img_batch, pts_batch, labels_batch, best_masks):
plt.figure(figsize=(10, 10))
plt.imshow(image)
for mask in masks:
show_mask(mask, plt.gca(), random_color=True)
show_points(points, labels, plt.gca())
好了,这篇文章就介绍到这里,后续还会继续更新,SAM2推理视频相关教程,感谢点赞关注!
资料获取
关于本文的相关代码及相关资料都已打包好,供需要的小伙伴们学习,获取方式如下:
更多推荐
所有评论(0)