I have an image containing text but with non straight lines drawn on it.
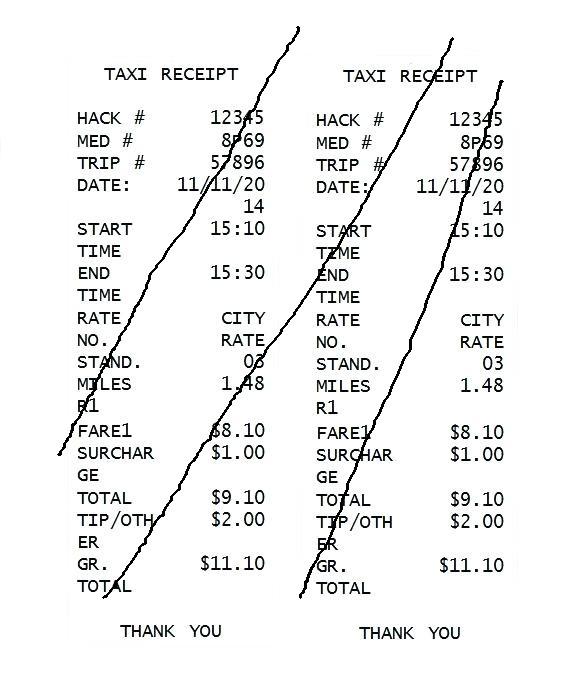
I want to remove those lines without affecting/removing anything from the text.
For that I used Hough probabilistic transform:
import cv2
import numpy as np
def remove_lines(filename):
img = cv2.imread(filename)
gray = cv2.cvtColor(img, cv2.COLOR_BGR2GRAY)
edges = cv2.Canny(gray, 50, 200)
lines = cv2.HoughLinesP(edges, rho=1, theta=1*np.pi/180,
threshold=100, minLineLength=100, maxLineGap=5)
# Draw lines on the image
for line in lines:
x1, y1, x2, y2 = line[0]
cv2.line(img, (x1, y1), (x2, y2), (0, 0, 255), 3)
cv2.imwrite('result', img)
The result was not as good as I expected:
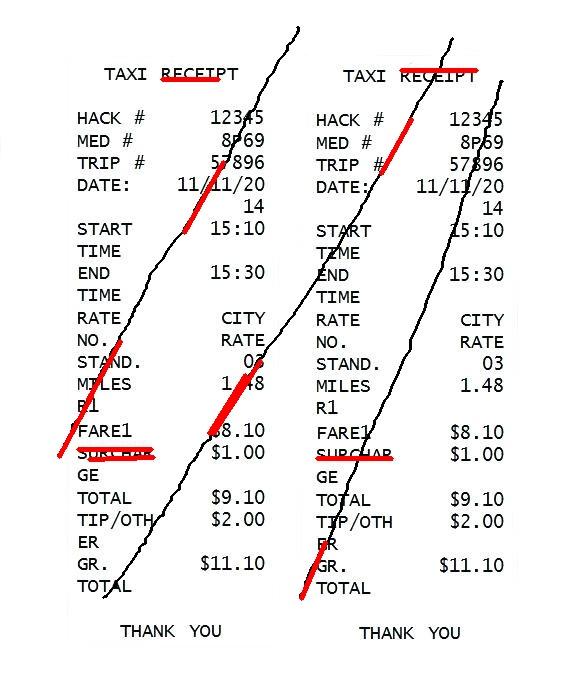
The lines were not entirely detected (only some segments, the straight segments, of the lines were detected).
I did some adjustments on cv2.Canny
and cv2.HoughLinesP
parameters, but it didn't work too.
I also tried cv2.createLineSegmentDetector
(Not available in the latest version of opencv due to license issue, so I had to downgrade opencv to version 4.0.0.21):
import cv2
import numpy as np
def remove_lines(filename):
im = cv2.imread(filename)
gray = cv2.cvtColor(im, cv2.COLOR_BGR2GRAY)
# Create default parametrization LSD
lsd = cv2.createLineSegmentDetector(0)
# Detect lines in the image (Position 0 of the returned tuple are the
# detected lines)
lines = lsd.detect(gray)[0]
# drawn_img = lsd.drawSegments(res, lines)
for element in lines:
if (abs(int(element[0][0]) - int(element[0][2])) > 70 or
abs(int(element[0][1]) - int(element[0][3])) > 70):
cv2.line(im, (int(element[0][0]), int(element[0][1])), (int(
element[0][2]), int(element[0][3])), (0, 0, 255), 3)
cv2.imwrite('lsd.jpg', im)
The result was a bit better, but didn't detect the entire lines.
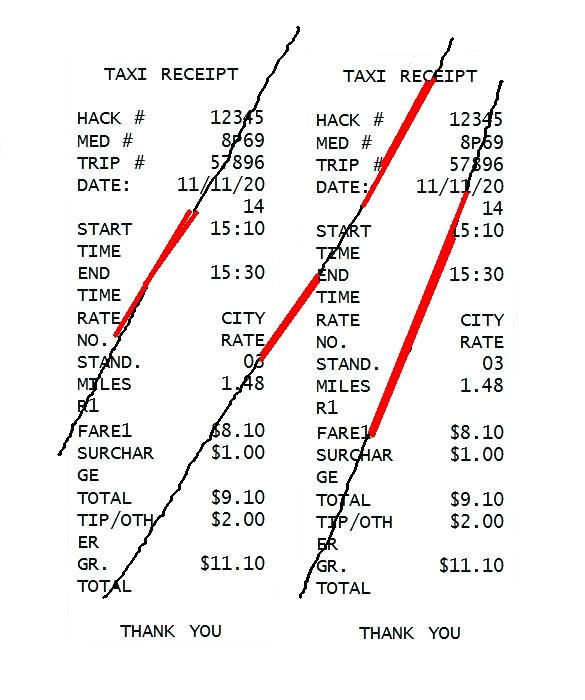
Any idea how to make the lines detection more efficient?
Typical methods to remove lines are to use horizontal/vertical kernels or cv2.HoughLinesP()
but these methods only work if the lines are straight. In this case, the lines are not straight so an idea is to use a diagonal kernel, morphological transformations, and contour filtering to remove the lines from the text. I will be using a previous answer's approach found in removing horizontal lines in an image but with a diagonal kernel
We begin by converting the image to grayscale and perform Otsu's threshold to obtain a binary image. Next we create a diagonal kernel then perform morph close to detect/filter out the diagonal lines. Since cv2.getStructuringElement()
does not have any built in diagonal kernel, we create our own
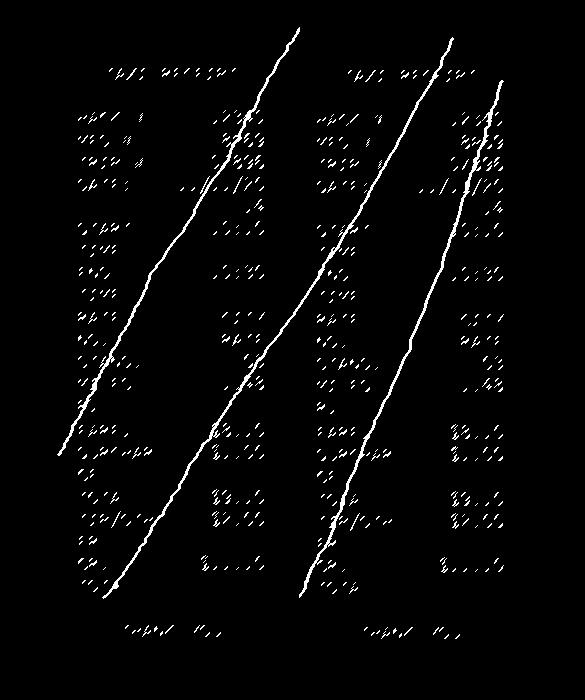
# Read in image, grayscale, and Otsu's threshold
image = cv2.imread('1.jpg')
gray = cv2.cvtColor(image, cv2.COLOR_BGR2GRAY)
thresh = cv2.threshold(gray, 0, 255,cv2.THRESH_BINARY_INV + cv2.THRESH_OTSU)[1]
# Create diagonal kernel
kernel = np.array([[0, 0, 1],
[0, 1, 0],
[1, 0, 0]], dtype=np.uint8)
opening = cv2.morphologyEx(thresh, cv2.MORPH_OPEN, kernel, iterations=1)
The image isolated the main diagonal lines but it also included small lines from the text. To remove them we find contours and filter using contour area. If the contour passes our filter, we effectively remove the noise by "filling in" the contour with cv2.drawContours()
. This leaves us with our desired diagonal lines to remove
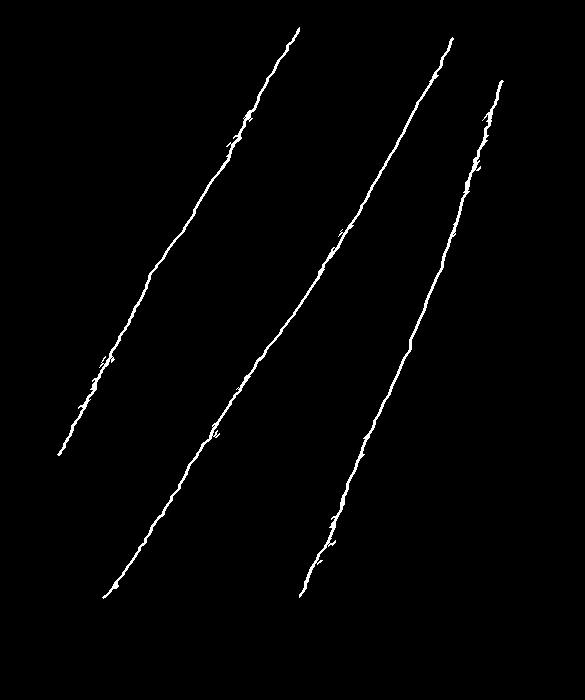
# Find contours and filter using contour area to remove noise
cnts = cv2.findContours(opening, cv2.RETR_EXTERNAL, cv2.CHAIN_APPROX_SIMPLE)
cnts = cnts[0] if len(cnts) == 2 else cnts[1]
for c in cnts:
area = cv2.contourArea(c)
if area < 500:
cv2.drawContours(opening, [c], -1, (0,0,0), -1)
From here we simply cv2.bitwise_xor()
with the original image to get our result
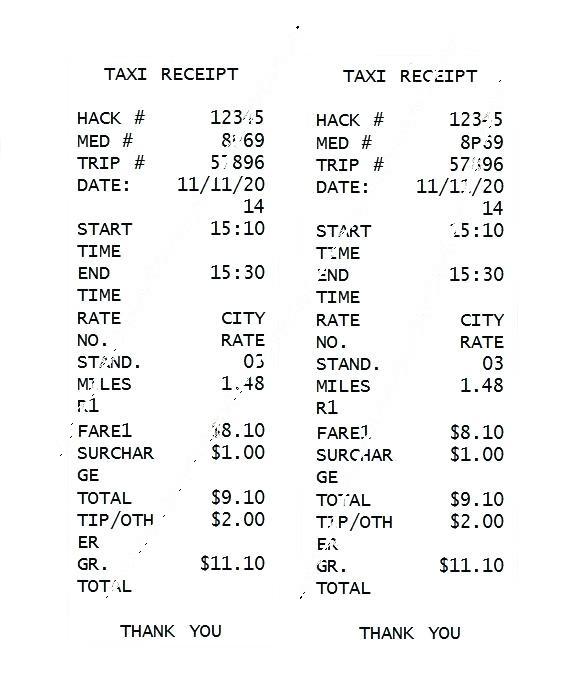
# Bitwise-xor with original image
opening = cv2.merge([opening, opening, opening])
result = cv2.bitwise_xor(image, opening)
Notes: It is difficult to remove the lines without affecting the text although it is possible and will need some clever tricks to "repair" the text. Take a look at remove borders from image but keep text written on borders for a method to reconstruct the missing text. Another method to isolate the diagonal lines would be to take a contrarian approach; instead of trying to detect diagnoal lines, why not try to determine what is not a diagnoal line. You could probably do this by simple filtering techniques. To create dynamic diagonal kernels, you could use np.diag()
for different diagonal line widths
Full code for completeness
import cv2
import numpy as np
# Read in image, grayscale, and Otsu's threshold
image = cv2.imread('1.jpg')
gray = cv2.cvtColor(image, cv2.COLOR_BGR2GRAY)
thresh = cv2.threshold(gray, 0, 255,cv2.THRESH_BINARY_INV + cv2.THRESH_OTSU)[1]
# Create diagonal kernel
kernel = np.array([[0, 0, 1],
[0, 1, 0],
[1, 0, 0]], dtype=np.uint8)
opening = cv2.morphologyEx(thresh, cv2.MORPH_OPEN, kernel, iterations=1)
# Find contours and filter using contour area to remove noise
cnts = cv2.findContours(opening, cv2.RETR_EXTERNAL, cv2.CHAIN_APPROX_SIMPLE)
cnts = cnts[0] if len(cnts) == 2 else cnts[1]
for c in cnts:
area = cv2.contourArea(c)
if area < 500:
cv2.drawContours(opening, [c], -1, (0,0,0), -1)
# Bitwise-xor with original image
opening = cv2.merge([opening, opening, opening])
result = cv2.bitwise_xor(image, opening)
cv2.imshow('thresh', thresh)
cv2.imshow('opening', opening)
cv2.imshow('result', result)
cv2.waitKey()
所有评论(0)