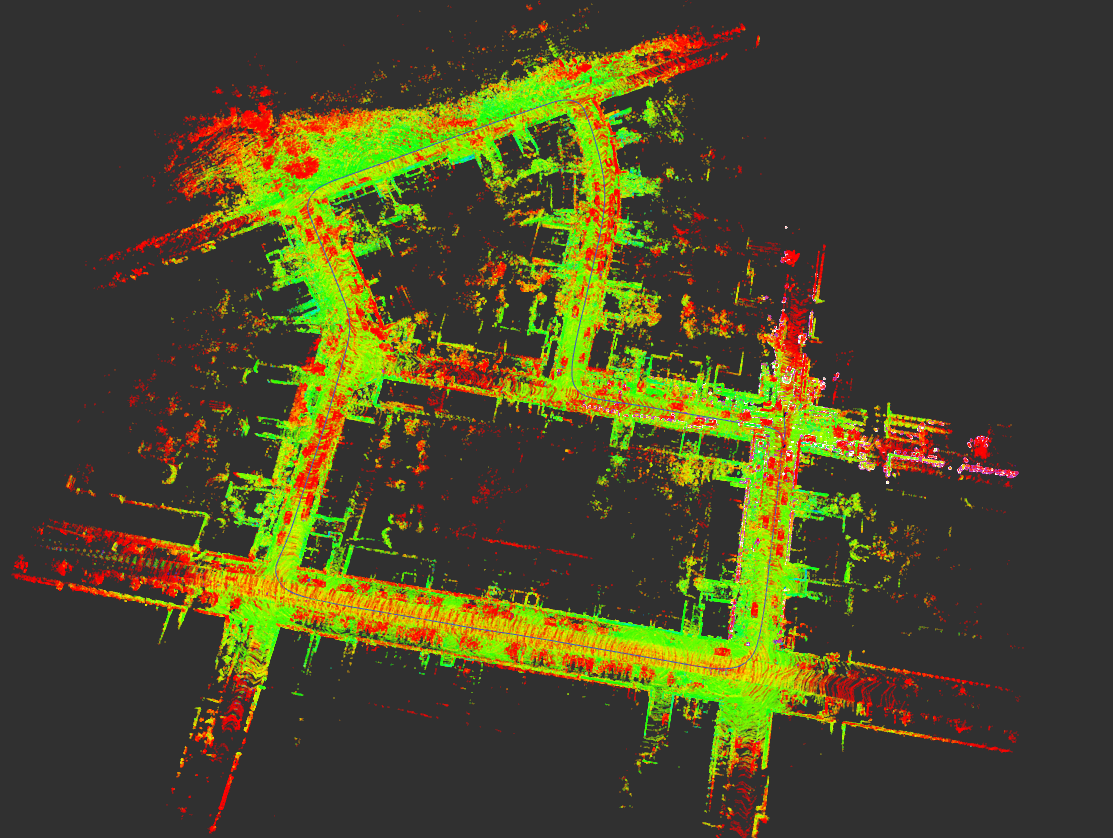
使用开源激光SLAM方案LIO-SAM运行KITTI数据集,如有用,请评论雷锋
LIOSAM运行KITTI数据集
第一次写博客~
-
LIOSAM作为优秀的激光slam方案,当然想尝试着运行更多数据啦,然而没有发现类似的方法分享到底如何实现,在B站看到有伙伴发布了测试视频,但是仍然没有写出方法。所以我跑通了之后记录一下
-
关于代码层面要修改的,因为liosam 要求输入的点云每个点都有ring 信息和相对时间信息,目前的雷达基本具备这些信息啦,但是早期的kitti数据集不具备,所以代码要自己计算一下 ring和time。方法可以参考lego-loam中这部分内容。多说一点,计算的时候每束激光都应该判断halfpassed,而lego-loam中只判断一次,我觉着是有点问题的,如果你不知道我在说什么,就先用lego-loam的方法修改就好了
-
关于数据,已有公开的kitti2bag 工具,使用方法:https://zhuanlan.zhihu.com/p/104875159 但是输出的bag liosam是不能正常跑的,位姿Z型突变,仔细了解一下发现 这个bag的imu频率跟雷达一样,也就是很低频。liosam作者提供了一个kitti bag 在google drive,至于如何下载就是另一个好问题 。 如果想自己制作bag, 作者自己改了kittitobag 就在源码的文件夹下,至少你还要多下载一个 *extract文件,举例如下:
然后运行 pip3 install tqdm
python3 kitti2bag.py -t 2011_09_30 -r 0027 raw_synced -
配置参数修改 只需要按照readme中修改:
extrinsicTrans: [-8.086759e-01, 3.195559e-01, -7.997231e-01]
extrinsicRot: [9.999976e-01, 7.553071e-04, -2.035826e-03, -7.854027e-04, 9.998898e-01, -1.482298e-02, 2.024406e-03, 1.482454e-02, 9.998881e-01]
extrinsicRPY: [9.999976e-01, 7.553071e-04, -2.035826e-03, -7.854027e-04, 9.998898e-01, -1.482298e-02, 2.024406e-03, 1.482454e-02, 9.998881e-01] -
是的 我想到了,您是不是觉着下载kitti再制作很麻烦, 想要我的bag呢,云盘如下:
请叫我雷锋
链接: https://pan.baidu.com/s/13MlLlzqUJME8ungRNJbaSQ 密码: hl71 -
运行结果:
备注:
我运行kitti的参数文件(部分):
# CPU Params
numberOfCores: 4 # number of cores for mapping optimization
mappingProcessInterval: 0.15 # seconds, regulate mapping frequency
# Topics for processing kitti by author
pointCloudTopic: "points_raw" # Point cloud data/ for kitti "/kitti/velo/pointcloud"
imuTopic: "imu_raw" # IMU data/ for kitti "/kitti/oxts/imu"
# Topics for normal kitti
# pointCloudTopic: "kitti/velo/pointcloud" # Point cloud data/ for kitti "/kitti/velo/pointcloud"
# imuTopic: "kitti/oxts/imu" # IMU data/ for kitti "/kitti/oxts/imu"
odomTopic: "odometry/imu" # IMU pre-preintegration odometry, same frequency as IMU
gpsTopic: "odometry/gpsz" # GPS odometry topic from navsat, see module_navsat.launch file
# Frames
lidarFrame: "base_link"
baselinkFrame: "base_link"
odometryFrame: "odom"
mapFrame: "map"
# GPS Settings
useImuHeadingInitialization: false # if using GPS data, set to "true"
useGPSInfo: false
useGpsElevation: false # if GPS elevation is bad, set to "false"
gpsCovThreshold: 2.0 # m^2, threshold for using GPS data
poseCovThreshold: 25.0 # m^2, threshold for using GPS data
# Export settings
savePCD: false # https://github.com/TixiaoShan/LIO-SAM/issues/3
savePCDDirectory: "/home/zch/00-test/SavedMap" # in your home folder, starts and ends with "/". Warning: the code deletes "LOAM" folder then recreates it. See "mapOptimization" for implementation
# Lidar Settings
# 特别注意: 如果 sensor: velodyne, useCloudRing 和useCloudTime 都为true!! 如果 sensor: kitti useCloudRing 和useCloudTime 都为false!!
useCloudRing: false
useCloudTime: false
sensor: kitti # lidar sensor type, either 'velodyne' or 'ouster' or kitti without ring information
N_SCAN: 64 # number of lidar channel (i.e., 16, 32, 64, 128)
Horizon_SCAN: 1800 # lidar horizontal resolution (Velodyne:1800, Ouster:512,1024,2048)
downsampleRate: 2 # default: 1. Downsample your data if too many points. i.e., 16 = 64 / 4, 16 = 16 / 1
lidarMinRange: 1.0 # default: 1.0, minimum lidar range to be used
lidarMaxRange: 250.0 # default: 1000.0, maximum lidar range to be used
lidar_has_ring: true
# IMU Settings
imuAccNoise: 3.9939570888238808e-03
imuGyrNoise: 1.5636343949698187e-03
imuAccBiasN: 6.4356659353532566e-05
imuGyrBiasN: 3.5640318696367613e-05
imuGravity: 9.80511
imuRPYWeight: 0.01
# Extrinsics (lidar -> IMU) for kitti
extrinsicTrans: [-8.086759e-01, 3.195559e-01, -7.997231e-01]
extrinsicRot: [9.999976e-01, 7.553071e-04, -2.035826e-03, -7.854027e-04, 9.998898e-01, -1.482298e-02, 2.024406e-03, 1.482454e-02, 9.998881e-01]
extrinsicRPY: [9.999976e-01, 7.553071e-04, -2.035826e-03, -7.854027e-04, 9.998898e-01, -1.482298e-02, 2.024406e-03, 1.482454e-02, 9.998881e-01]
# LOAM feature threshold
edgeThreshold: 1.0
surfThreshold: 0.1
edgeFeatureMinValidNum: 10
surfFeatureMinValidNum: 100
# voxel filter paprams
odometrySurfLeafSize: 0.4 # default: 0.4 - outdoor, 0.2 - indoor
mappingCornerLeafSize: 0.2 # default: 0.2 - outdoor, 0.1 - indoor
mappingSurfLeafSize: 0.4 # default: 0.4 - outdoor, 0.2 - indoor
# robot motion constraint (in case you are using a 2D robot)
z_tollerance: 1000 # meters
rotation_tollerance: 1000 # radians
# Surrounding map
surroundingkeyframeAddingDistThreshold: 1.0 # meters, regulate keyframe adding threshold
surroundingkeyframeAddingAngleThreshold: 0.2 # radians, regulate keyframe adding threshold
surroundingKeyframeDensity: 2.0 # meters, downsample surrounding keyframe poses
surroundingKeyframeSearchRadius: 50.0 # meters, within n meters scan-to-map optimization (when loop closure disabled)
更多推荐
所有评论(0)