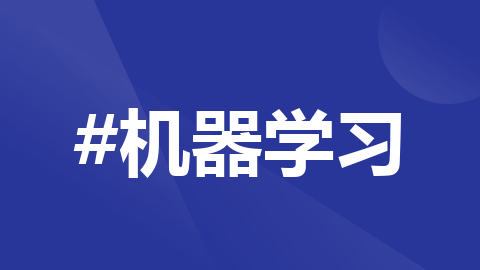
脉冲神经网络(SNN)概述
脉冲神经网络(SNN)作为一种具有二元脉冲信息传递机制、丰富时空动态和事件驱动特征的脑启发计算模型,受到了广泛的关注。但其复杂的不连续脉冲机制给深度信噪网络的优化带来了困难。由于代理梯度方法可以极大地降低优化难度,在直接训练深度SNN方面显示出巨大的潜力,近年来,各种基于直接学习的深度SNN工作被提出,并取得了令人满意的进展。主要分为精度提高方法、效率提高方法和时间动态利用方法。
目录
前言
脉冲神经网络(SNN)作为一种具有二元脉冲信息传递机制、丰富时空动态和事件驱动特征的脑启发计算模型,受到了广泛的关注。但其复杂的不连续脉冲机制给深度信噪网络的优化带来了困难。由于代理梯度方法可以极大地降低优化难度,在直接训练深度SNN方面显示出巨大的潜力,近年来,各种基于直接学习的深度SNN工作被提出,并取得了令人满意的进展。主要分为精度提高方法、效率提高方法和时间动态利用方法。
一、SNN特点
1.1 丰富的时间动态
神经元模型:
其中表示第l层在t时间的膜电位,
是时间泄漏常数,取值范围(0,1),
是权重矩阵,
表示前一层在t时间的输出,
是发射阈值。 由式子可知,与人工神经网络不同,SNN在峰值神经元模型中具有独特的时空动态特性。
当膜电位超过发射阈值时,它将发射一个尖峰,然后下降到静息电位:
1.2 效率高
由于输出是二进制张量,激活和权重的乘法可以用加法代替,因此具有很高的能量效率。此外,当 没有产生脉冲输出时,神经元将保持在静息电位。在神经形态硬件中实现时,这种事件驱动机制可以进一步节省能量。
1.3 代表能力有限
通过将实值膜电位量化为二值输出脉冲来传输信息会在SNN中引入量化误差,从而造成信息损失,此外,来自时间步长的二进制脉冲特征映射不能像人工神经网络中的实值特征映射那样携带足够的信息。这两个问题在一定程度上限制了SNN的表征能力。
1.4 激发函数的不可微性
由于SNN的发射过程是不可微的,因此无法通过基于梯度的优化方法训练SNN,因此训练深度SNN是一个挑战。
二、已有的改进
2.1 精度改进方法
2.1.1 提高代表能力
- 在神经元层面:使脉冲神经元中的一些超参数可学习[1]、采用可学习膜时间常数[2]。
- 在网络结构层面:改进SNN架构[3],引入可学习注意机制[4][5],训练推理解耦结构[6]。
- 在训练技术层面:使用正则化[7]和蒸馏技术[8]。
2.1.2 缓解训练困难
对脉冲神经元使用替代梯度(SG)函数,SG方法利用可微的替代函数代替不可微的发射活动来计算反向传播中的梯度,可以缓解不可微性问题,但发射函数的梯度与替代梯度之间存在明显的梯度不匹配。这个问题很容易导致SNN优化不足,导致严重的性能下降。此外,由于大多数SG方法采用了类tanh函数,SNN中的梯度爆炸/消失问题比ANN更严重。
为了解决这些问题,提出了以下方法:
- 设计更好的SG方法:基于有限差分技术的可微分脉冲方法[9]、可微SG搜索(DGS)方法[10]。
- 缓解梯度爆炸/消失问题:使用改进的神经元或架构[3][11],改进的批归一化和正则化[12]。
2.2 效率改进方法
2.2.1 网络压缩技术
- 参数修建:通过去除不重要的参数来消除模型中的冗余参数提高效率[13]。
- 神经结构搜索(NAS):使用NAS技术让算法自动设计紧凑的神经结构[14]。
- 知识蒸馏:从一个大的模型中得到一个紧凑的模型[15]。
2.2.2 稀疏SNN
SNN通过脉冲事件传递信息,只有当神经元接收到尖峰事件时才会进行计算。得益于这种事件驱动的计算机制,SNN在神经形态硬件上实现时可以大大节省能量并高效运行。因此,限制峰值神经元的放电速率来实现稀疏SNN也是一种广泛使用的提高SNN效率的方法[16]。
2.3 时间动态利用方法
2.3.1 顺序学习
在许多工作中,SNN在顺序学习上表现出比具有相似尺度的ann更好的性能[17]。
2.3.2 结合事件相机
事件相机由于能够异步捕获时间丰富的信息,最近在高速运动估计中显示出巨大的潜力,SNN具有时空和事件驱动的处理机制,非常适合处理这种异步数据。许多优秀的作品结合SNN和神经形态相机来解决现实世界的大规模问题[18]。
参考文献
[1] Bellec, G., Salaj, D., Subramoney, A., Legenstein, R., and Maass, W. (2018). Long short-term memory and learning-to-learn in networks of spiking neurons. Advances in neural information processing systems 31
[2] Yin, B., Corradi, F., and Bohte, S. M. (2020). Effective and efficient computation with multiple-timescale spiking recurrent neural networks. In International Conference on Neuromorphic Systems 2020. 1–8
[3] Fang, W., Yu, Z., Chen, Y., Huang, T., Masquelier, T., and Tian, Y. (2021a). Deep residual learning in spiking neural networks. Advances in Neural Information Processing Systems 34, 21056–21069
[4] Yao, M., Gao, H., Zhao, G., Wang, D., Lin, Y., Yang, Z., et al. (2021). Temporal-wise attention
spiking neural networks for event streams classification. In Proceedings of the IEEE/CVF International Conference on Computer Vision. 10221–10230
[5] Yu, C., Gu, Z., Li, D., Wang, G., Wang, A., and Li, E. (2022a). Stsc-snn: Spatio-temporal synaptic connection with temporal convolution and attention for spiking neural networks. arXiv preprint arXiv:2210.05241
[6] Guo, Y., Zhang, L., Chen, Y., Tong, X., Liu, X., Wang, Y., et al. (2022d). Real spike: Learning real-valued spikes for spiking neural networks. In Computer Vision–ECCV 2022: 17th European Conference, Tel Aviv, Israel, October 23–27, 2022, Proceedings, Part XII (Springer), 52–68
[7] Guo, Y., Chen, Y., Zhang, L., Liu, X., Wang, Y., Huang, X., et al. (2022a). IM-loss: Information
maximization loss for spiking neural networks. In Advances in Neural Information Processing Systems, eds. A. H. Oh, A. Agarwal, D. Belgrave, and K. Cho
[8] Kushawaha, R. K., Kumar, S., Banerjee, B., and Velmurugan, R. (2021). Distilling spikes: Knowledge distillation in spiking neural networks. In 2020 25th International Conference on Pattern Recognition (ICPR) (IEEE), 4536–4543
[9] Li, Y., Guo, Y., Zhang, S., Deng, S., Hai, Y., and Gu, S. (2021b). Differentiable spike: Rethinkinggradient-descent for training spiking neural networks. Advances in Neural Information Processing Systems 34, 23426–23439
[10] Leng, L., Che, K., Zhang, K., Zhang, J., Meng, Q., Cheng, J., et al. (2022). Differentiable hierarchical and surrogate gradient search for spiking neural networks. In Advances in Neural Information Processing Systems, eds. A. H. Oh, A. Agarwal, D. Belgrave, and K. Cho
[11] Zhang, M., Wang, J., Wu, J., Belatreche, A., Amornpaisannon, B., Zhang, Z., et al. (2022c). Rectified linear postsynaptic potential function for backpropagation in deep spiking neural networks. IEEE Transactions on Neural Networks and Learning Systems 33, 1947–1958. doi:10.1109/TNNLS.2021.3110991
[12] Zheng, H., Wu, Y., Deng, L., Hu, Y., and Li, G. (2021). Going deeper with directly-trained larger spiking neural networks. In Proceedings of the AAAI Conference on Artificial Intelligence. vol. 35, 11062–11070
[13] Chowdhury, S. S., Garg, I., and Roy, K. (2021). Spatio-temporal pruning and quantization for low-latency spiking neural networks. In 2021 International Joint Conference on Neural Networks (IJCNN) (IEEE),1–9
[14] Na, B., Mok, J., Park, S., Lee, D., Choe, H., and Yoon, S. (2022). Autosnn: Towards energy-efficient spiking neural networks. arXiv preprint arXiv:2201.12738
[15] Kushawaha, R. K., Kumar, S., Banerjee, B., and Velmurugan, R. (2021). Distilling spikes: Knowledgedistillation in spiking neural networks. In 2020 25th International Conference on Pattern Recognition (ICPR) (IEEE), 4536–4543
[16] Zambrano, D. and Bohte, S. M. (2016). Fast and efficient asynchronous neural computation with adapting spiking neural networks. arXiv preprint arXiv:1609.02053
[17] Wu, J., Yılmaz, E., Zhang, M., Li, H., and Tan, K. (2020). Deep spiking neural networks for large vocabulary automatic speech recognition. Frontiers in Neuroscience 14. doi:10.3389/fnins.2020.00199
[18] Gao, Y., Li, S., Li, Y., Guo, Y., and Dai, Q. (2022). Superfast: 200x video frame interpolation via event camera. IEEE Transactions on Pattern Analysis and Machine Intelligence
更多推荐
所有评论(0)