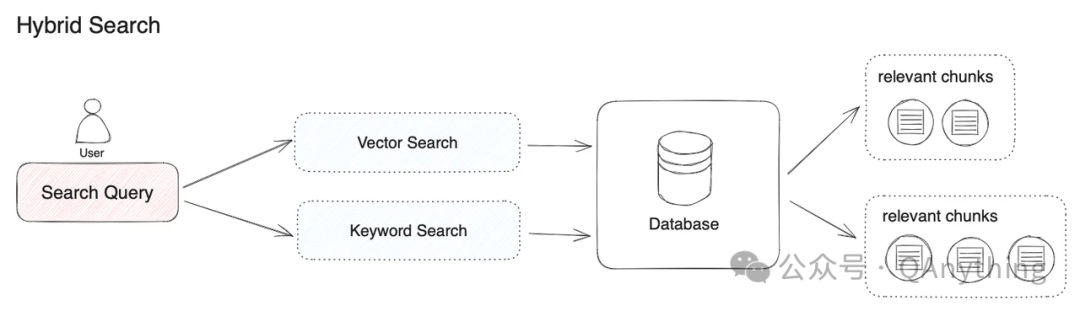
QAnything-1.3.0,支持纯python笔记本运行,支持混合检索
QAnything 1.3.0 更新了,这次带来两个主要功能,一个是纯python的安装,另一个是混合检索。更多详情见:我们刚发布qanything开源的时候,希望用户可以用这个代码来直接在生产环境中部署使用,为了性能,它引入了很多第三方的库和服务,比如milvus,mysql,tritonserver,elasticsearch等。这些服务本身也非常庞大复杂,我们做了docker镜像和docke
QAnything 1.3.0 更新了,这次带来两个主要功能,一个是纯python的安装,另一个是混合检索。更多详情见:
https://github.com/netease-youdao/QAnything/releases
纯python安装
我们刚发布qanything开源的时候,希望用户可以用这个代码来直接在生产环境中部署使用,为了性能,它引入了很多第三方的库和服务,比如milvus,mysql,tritonserver,elasticsearch等。这些服务本身也非常庞大复杂,我们做了docker镜像和dockerfiles,试图将一些依赖打包起来,用户只要拉下来就可以用。但是还是有很多人遇到麻烦。比如不能在mac等笔记本上运行。
所以这次,这次我们发布了一个纯python的轻量级的版本,可以在mac等笔记本上跑起来,可以不依赖gpu。安装过程极其简单:
第一步:拉代码到本地
git clone https://github.com/netease-youdao/QAnything
如果是国内的访问不了github的,可以用gitee,我们已经同步了代码。
git clone https://gitee.com/netease-youdao/QAnything.git
第二步:安装
首先需要将代码分支切换到develop_for_v1.3.1的版本(因为这个版本还在开发中),然后执行安装:
cd QAnything
git checkout develop_for_v1.3.1
pip install -e .
在这一步,系统会自动检测所有依赖的东西,包括vllm,transformer,pytorch等库。为了提高国内用户的下载速度,里面大部分的源都已经针对国内环境做了优化。
注意,如果是Mac下,需要先安装xcode(在mac app store上可以找到),因为它依赖了lamma.cpp,需要编译一下。
第三步:使用
为了方便用户使用,我们在scripts下面放了针对多个机器环境配置的一键启动的脚本:
ls scripts/
base_run.sh run_for_4B_in_M1_mac.sh
gpu_capabilities.json run_for_7B_in_Linux_or_WSL.sh
list_files.py run_for_7B_in_M1_mac.sh
local_chat_qa.py run_for_openai_api_in_M1_mac.sh
multi_local_chat_qa.py run_for_openai_api_with_cpu_in_Linux_or_WSL.sh
multi_upload_files.py run_for_openai_api_with_gpu_in_Linux_or_WSL.sh
mysql_statics.py stream_chat.py
new_knowledge_base.py upload_files.py
nvidia_gpus_compute_capability.py weixiaobao.jpg
run_for_3B_in_Linux_or_WSL.sh
以笔者的电脑为例(mac m1),我可以选择用:
scripts/run_for_openai_api_in_M1_mac.sh
这个脚本使用了本地的embedding/rerank/ocr,但是得配置一下openai的接口。这个需要大家找一下,有很多代理openai的接口,可以配置baseurl和key。
假设我们已经配置好了,就可以通过这个脚本(我隐去了里面的url和key,用*替代)启动qanything了:
(base) mac:QAnything linhui$ sh scripts/run_for_openai_api_in_M1_mac.sh
Xcode 已正确安装在路径:/Applications/Xcode.app/Contents/Developer
即将启动后端服务,启动成功后请复制[http://127.0.0.1:8777/qanything/]到浏览器进行测试。
运行qanything-server的命令是:
python3 -m qanything_kernel.qanything_server.sanic_api --host 127.0.0.1 --port 8777 --model_size 7B --use_openai_api --openai_api_base https://api.openai****.org/v1 --openai_api_key sk-AM3*************BHl --openai_api_model_name gpt-3.5-turbo-1106 --openai_api_context_length 4096 --workers 4
LOCAL DATA PATH: /Users/linhui/workspace/QAnything/QANY_DB/content
LOCAL_RERANK_REPO: maidalun/bce-reranker-base_v1
LOCAL_EMBED_REPO: maidalun/bce-embedding-base_v1
<Logger debug_logger (INFO)> <Logger qa_logger (INFO)>
llama_cpp_python 0.2.57 已经安装。
2024-04-08 10:03:13,141 - modelscope - INFO - PyTorch version 2.2.1 Found.
2024-04-08 10:03:13,141 - modelscope - INFO - Loading ast index from /Users/linhui/.cache/modelscope/ast_indexer
2024-04-08 10:03:13,176 - modelscope - INFO - Loading done! Current index file version is 1.13.0, with md5 4e15c4f2db78c84e863a425f008f4eac and a total number of 972 components indexed
use_cpu: False
use_openai_api: True
[2024-04-08 10:03:14 +0800] [20092] [INFO]
┌─────────────────────────────────────────────────────────────────────────────┐
│ Sanic v23.6.0 │
│ Goin' Fast @ http://127.0.0.1:8777 │
├───────────────────────┬─────────────────────────────────────────────────────┤
│ │ mode: production, w/ 4 workers │
│ ▄███ █████ ██ │ server: sanic, HTTP/1.1 │
│ ██ │ python: 3.10.9 │
│ ▀███████ ███▄ │ platform: macOS-13.3.1-arm64-arm-64bit │
│ ██ │ packages: sanic-routing==23.12.0, sanic-ext==23.6.0 │
│ ████ ████████▀ │ │
│ │ │
│ Build Fast. Run Fast. │ │
└───────────────────────┴─────────────────────────────────────────────────────┘
[2024-04-08 10:03:14 +0800] [20092] [WARNING] Sanic is running in PRODUCTION mode. Consider using '--debug' or '--dev' while actively developing your application.
LOCAL DATA PATH: /Users/linhui/workspace/QAnything/QANY_DB/content
LOCAL_RERANK_REPO: maidalun/bce-reranker-base_v1
LOCAL_EMBED_REPO: maidalun/bce-embedding-base_v1
<Logger debug_logger (INFO)> <Logger qa_logger (INFO)>
llama_cpp_python 0.2.57 已经安装。
2024-04-08 10:03:17,231 - modelscope - INFO - PyTorch version 2.2.1 Found.
2024-04-08 10:03:17,231 - modelscope - INFO - Loading ast index from /Users/linhui/.cache/modelscope/ast_indexer
2024-04-08 10:03:17,264 - modelscope - INFO - Loading done! Current index file version is 1.13.0, with md5 4e15c4f2db78c84e863a425f008f4eac and a total number of 972 components indexed
use_cpu: False
use_openai_api: True
LOCAL DATA PATH: /Users/linhui/workspace/QAnything/QANY_DB/content
LOCAL_RERANK_REPO: maidalun/bce-reranker-base_v1
LOCAL_EMBED_REPO: maidalun/bce-embedding-base_v1
<Logger debug_logger (INFO)> <Logger qa_logger (INFO)>
LOCAL DATA PATH: /Users/linhui/workspace/QAnything/QANY_DB/content
LOCAL_RERANK_REPO: maidalun/bce-reranker-base_v1
LOCAL_EMBED_REPO: maidalun/bce-embedding-base_v1
<Logger debug_logger (INFO)> <Logger qa_logger (INFO)>
LOCAL DATA PATH: /Users/linhui/workspace/QAnything/QANY_DB/content
LOCAL_RERANK_REPO: maidalun/bce-reranker-base_v1
LOCAL_EMBED_REPO: maidalun/bce-embedding-base_v1
LOCAL DATA PATH: /Users/linhui/workspace/QAnything/QANY_DB/content
LOCAL_RERANK_REPO: maidalun/bce-reranker-base_v1
LOCAL_EMBED_REPO: maidalun/bce-embedding-base_v1
<Logger debug_logger (INFO)> <Logger qa_logger (INFO)>
<Logger debug_logger (INFO)> <Logger qa_logger (INFO)>
llama_cpp_python 0.2.57 已经安装。
llama_cpp_python 0.2.57 已经安装。
llama_cpp_python 0.2.57 已经安装。
llama_cpp_python 0.2.57 已经安装。
2024-04-08 10:03:21,898 - modelscope - INFO - PyTorch version 2.2.1 Found.
2024-04-08 10:03:21,898 - modelscope - INFO - PyTorch version 2.2.1 Found.
2024-04-08 10:03:21,898 - modelscope - INFO - Loading ast index from /Users/linhui/.cache/modelscope/ast_indexer
2024-04-08 10:03:21,898 - modelscope - INFO - Loading ast index from /Users/linhui/.cache/modelscope/ast_indexer
2024-04-08 10:03:21,947 - modelscope - INFO - Loading done! Current index file version is 1.13.0, with md5 4e15c4f2db78c84e863a425f008f4eac and a total number of 972 components indexed
2024-04-08 10:03:21,951 - modelscope - INFO - Loading done! Current index file version is 1.13.0, with md5 4e15c4f2db78c84e863a425f008f4eac and a total number of 972 components indexed
2024-04-08 10:03:22,335 - modelscope - INFO - PyTorch version 2.2.1 Found.
2024-04-08 10:03:22,336 - modelscope - INFO - Loading ast index from /Users/linhui/.cache/modelscope/ast_indexer
2024-04-08 10:03:22,369 - modelscope - INFO - Loading done! Current index file version is 1.13.0, with md5 4e15c4f2db78c84e863a425f008f4eac and a total number of 972 components indexed
2024-04-08 10:03:22,431 - modelscope - INFO - PyTorch version 2.2.1 Found.
2024-04-08 10:03:22,432 - modelscope - INFO - Loading ast index from /Users/linhui/.cache/modelscope/ast_indexer
2024-04-08 10:03:22,466 - modelscope - INFO - Loading done! Current index file version is 1.13.0, with md5 4e15c4f2db78c84e863a425f008f4eac and a total number of 972 components indexed
use_cpu: False
use_openai_api: True
use_cpu: False
use_openai_api: True
[2024-04-08 10:03:22 +0800] [20102] [INFO] Sanic Extensions:
[2024-04-08 10:03:22 +0800] [20102] [INFO] > injection [0 dependencies; 0 constants]
[2024-04-08 10:03:22 +0800] [20102] [INFO] > openapi [http://127.0.0.1:8777/docs]
[2024-04-08 10:03:22 +0800] [20102] [INFO] > http
[2024-04-08 10:03:22 +0800] [20102] [INFO] > templating [jinja2==3.1.2]
[2024-04-08 10:03:22 +0800] [20099] [INFO] Sanic Extensions:
[2024-04-08 10:03:22 +0800] [20099] [INFO] > injection [0 dependencies; 0 constants]
[2024-04-08 10:03:22 +0800] [20099] [INFO] > openapi [http://127.0.0.1:8777/docs]
[2024-04-08 10:03:22 +0800] [20099] [INFO] > http
[2024-04-08 10:03:22 +0800] [20099] [INFO] > templating [jinja2==3.1.2]
use_cpu: False
use_openai_api: True
[2024-04-08 10:03:23 +0800] [20101] [INFO] Sanic Extensions:
[2024-04-08 10:03:23 +0800] [20101] [INFO] > injection [0 dependencies; 0 constants]
[2024-04-08 10:03:23 +0800] [20101] [INFO] > openapi [http://127.0.0.1:8777/docs]
[2024-04-08 10:03:23 +0800] [20101] [INFO] > http
[2024-04-08 10:03:23 +0800] [20101] [INFO] > templating [jinja2==3.1.2]
use_cpu: False
use_openai_api: True
[2024-04-08 10:03:23 +0800] [20100] [INFO] Sanic Extensions:
[2024-04-08 10:03:23 +0800] [20100] [INFO] > injection [0 dependencies; 0 constants]
[2024-04-08 10:03:23 +0800] [20100] [INFO] > openapi [http://127.0.0.1:8777/docs]
[2024-04-08 10:03:23 +0800] [20100] [INFO] > http
[2024-04-08 10:03:23 +0800] [20100] [INFO] > templating [jinja2==3.1.2]
然后就可以通过浏览器访问:http://127.0.0.1:8777/qanything/#/home 来体验qanything了。
当然,也可以使用本地的大模型,qanything已经通过lamma.cpp以及vllm 集成了多个本地大模型。在mac的笔记本上是千问7b和4b的int4的量化版本,我测试了感觉效果一般。在linux + nvidia显卡上用的是千问7b的int8的版本,效果还行。
目前python版(develop_v1.3.1分支)相比docker版本(master 分支)在易用性上有优势,但是一些性能相关的东西还没有迁移过来,比如docker版本支持tensortllm,推理性能更高一些。docker版本里面的ocr解析模型质量也略好点。
混合检索
这次我们同时支持了混合检索 BM25 + embedding。
embedding语义检索
qanything自带了bcembedding的模型,可以做语义检索,大部分情况下已经能取得比较好的效果。
BM25关键字搜索
在实际使用中,我们发现有一些特殊情况用关键字搜索效果会更好,包括:
-
特定名字的搜索。比如iphone 15。如果用向量检索可能会把所有的iphone都给检索出来了,而我只要iphone 15这个型号的。
-
罕见的短词、缩略词搜索。这个因为训练语料中见的很少,可能会导致语义理解发生偏差,检索不出来。
-
一些id的检索。需要做精确匹配的。
BM25算法的解释
BM25算法是比较经典的关键字搜索算法,它是由tf/idf算法改进而来的。2016年Elasticsearch 5.0的默认检索器从tf/idf改成了bm25算法。这里简单科普下这个算法:
tf/idf算法
搜索query和doc的匹配度的score计算公式为:
score(D, T) = tf * idf = termFrequency(D, T) * log(N / docFrequency(T))
其中 termFrequency 是词频,代表query的词在某个文档中出现的次数,出现的越多次就越相关。idf代表这个词的区分度,如果一个词(比如“的”、“地”、“得”之类副词)在很多文档都出现,这种词就不太重要。N是文档总数,docFrequency代表这个词在多少个文档中出现。其中Log是为了平滑,否则细微的docFrequency的变化会导致score的剧烈波动。
BM25算法
bm25算法在tf/idf算法上做了几点改进:
1.词频贡献有个上限。比如一个文档出现了太多次query里面的某个词了,这个贡献不应一直累加,而是有个上限。所以它用tf/(tf+k) 来做抑制。这带来的一个好处是,算法会青睐不同query的词同时在文档中出现的情况。比如,搜索 cat dog,如果cat 和 dog都在文档中出现1次,它的分值会比只有dog在文档中出现2次的分值要高。如果k=1的话,一个词出现两次的score=1/2,两个词出现1次的socre = 2/3。
2. 惩罚长文档的影响力。如果文档词比较多,那么命中关键字的可能性当然就大很多。把 |D|/(avg doc length)作为k,可以达到这个目的。
3. 词的重要性。这个还是按idf的那套策略来算。这里用的是log (N-DF+.5)/(DF+.5),这是由学术理论算出来的最优值(在一些简化的假设条件下)。
重排序(rerank)
两路检索出来的的chunks要按照重要性做重新排序。重排序也有多种算法,比如基于倒数的融合排序:
不过在我们系统中,我们直接依赖BCE rerank做重排序,在各种场景下表现良好。
混合检索当前还只在docker版本(master 分支) 上支持。
qanything 1.3.0的更新
新特性
-
支持在纯Python环境中安装,可以在MAC上运行,也可以在纯CPU机器上运行。详见:纯Python环境安装教程
-
支持混合检索(BM25+Embedding)。
-
当系统命令缺失时,下载提示更清晰。
-
自动检测显卡的计算能力和内存大小,并根据情况自动调整默认启动参数,以提供用户最佳体验,并给予相关提示。
-
更新前置条件,最低支持 GTX1050Ti,支持更多显卡型号。
-
提示用户代码仓库是否为最新,仅适用于主分支。
-
优化启动流程,自动查询相关日志并在前端显示错误消息,避免连续输出 'waiting for coffee'。
-
在前端添加英文版本。
-
修复已知的错误,优化用户体验。(在 Milvus 插入失败时,请记得删除根目录下的 "volumes" 文件夹。)
变更内容
-
修复启动脚本已知问题。由 @xixihahaliu 提交于 #92
-
修复已知问题,优化用户体验。由 @xixihahaliu 提交于 #102
-
Milvus增加用户名、密码、数据库名支持。由 @cklogic 提交于 #97
-
feat_#114: 为 NVIDIA GeForce RTX 4090D 添加 GPU 兼容性检查。由 @johnymoo 提交于 #115
-
修复:由 @pinkcxy 提交的轮次控制问题。#131
-
问题修复:调用api/api/local_doc_qa/local_doc_chat返回数据为None。由 @leehom0123 提交于 #137
-
优化解析 csv 和 xlsx 文件的逻辑。此外,现在支持离线运行 Docker 镜像。由 @xixihahaliu 提交于 #139
-
混合搜索。由 @shenlei1020 提交于 #194
QAnything开源代码地址:
https://github.com/netease-youdao/QAnything
更多信息见:
-
纯python的安装说明:https://github.com/netease-youdao/QAnything/blob/qanything-python-v1.3.1/README.md#pure-python-environment-installation-guide
-
bce embedding 和 rerank: https://github.com/netease-youdao/BCEmbedding
-
bm25算法的解释:https://kmwllc.com/index.php/2020/03/20/understanding-tf-idf-and-bm-25/
更多推荐
所有评论(0)