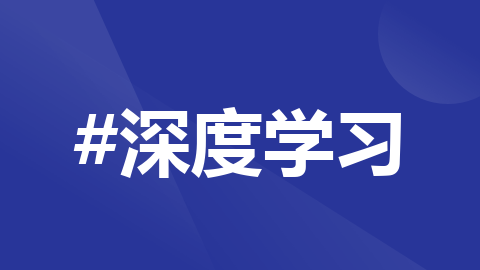
深度学习自动调参--optuna库
【代码】深度学习自动调参--optuna库。
介绍
Optuna 是一个开源的自动超参数优化软件库,它的原理基于以下关键特征:
-
轻量化、多功能及平台无关的架构:Optuna 被设计为易于安装和部署在不同的环境中,不受限于特定的平台或技术栈1。
-
Pythonic搜索空间:Optuna 允许用户以直观的Python代码定义搜索空间,使得定义和修改超参数的过程变得简单和自然1。
-
高效的优化算法:Optuna 实现了一系列高效的算法来优化超参数,例如贝叶斯优化、CMA-ES以及随机搜索1。
-
易于并行化:Optuna 设计以支持易于设置的并行化超参数搜索,从而加速实验过程1。
-
快速可视化超参数优化分析:Optuna 提供了快速可视化工具,帮助用户分析和理解超参数优化过程1。
以上特征使得Optuna 在自动化和加速机器学习模型的超参数调整过程中非常有效。
示例
import optuna
import torch
import torch.nn as nn
import torch.optim as optim
import torch.nn.functional as F
from torchvision import datasets, transforms
from torch.utils.data import DataLoader
# 定义模型
class SimpleMLP(nn.Module):
def __init__(self, input_size, hidden_size, output_size):
super(SimpleMLP, self).__init__()
self.fc1 = nn.Linear(input_size, hidden_size)
self.fc2 = nn.Linear(hidden_size, output_size)
def forward(self, x):
x = F.relu(self.fc1(x))
x = F.log_softmax(self.fc2(x), dim=1)
return x
# 使用Optuna定义一个目标函数进行优化
def objective(trial):
# 加载MNIST数据
transform = transforms.ToTensor()
train_dataset = datasets.MNIST(root="./data", train=True, transform=transform, download=True)
train_loader = DataLoader(train_dataset, batch_size=128, shuffle=True)
# 为超参数定义搜索空间
lr = trial.suggest_float("lr", 1e-5, 1e-1, log=True)
hidden_size = trial.suggest_int("hidden_size", 32, 512, log=True)
model = SimpleMLP(input_size=28 * 28, hidden_size=hidden_size, output_size=10)
optimizer = optim.Adam(model.parameters(), lr=lr)
criterion = nn.NLLLoss()
device = torch.device("cuda" if torch.cuda.is_available() else "cpu")
model.to(device)
# 训练模型
for epoch in range(3): # 使用3个周期仅作为示例
model.train()
for batch_idx, (data, target) in enumerate(train_loader):
data, target = data.to(device), target.to(device)
data = data.view(data.size(0), -1)
optimizer.zero_grad()
output = model(data)
loss = criterion(output, target)
loss.backward()
optimizer.step()
# 返回最后一个周期的损失值作为目标值
return loss.item()
# 使用Optuna进行优化
study = optuna.create_study(direction="minimize")
study.optimize(objective, n_trials=10)
# 输出最佳结果
print(f"The best parameters are {study.best_params} with a loss of {study.best_value}.")
输出结果
[I 2023-10-28 20:17:50,511] Trial 0 finished with value: 0.04887419566512108 and parameters: {'lr': 0.011838300770939164, 'hidden_size': 77}. Best is trial 0 with value: 0.04887419566512108.
[I 2023-10-28 20:18:11,788] Trial 1 finished with value: 0.4912452697753906 and parameters: {'lr': 9.935485179439176e-05, 'hidden_size': 34}. Best is trial 0 with value: 0.04887419566512108.
[I 2023-10-28 20:18:32,769] Trial 2 finished with value: 1.0512008666992188 and parameters: {'lr': 1.4223589989354732e-05, 'hidden_size': 132}. Best is trial 0 with value: 0.04887419566512108.
[I 2023-10-28 20:18:53,770] Trial 3 finished with value: 0.01927812770009041 and parameters: {'lr': 0.007958220298373548, 'hidden_size': 115}. Best is trial 3 with value: 0.01927812770009041.
[I 2023-10-28 20:19:15,002] Trial 4 finished with value: 0.36335524916648865 and parameters: {'lr': 0.0001498928287332272, 'hidden_size': 136}. Best is trial 3 with value: 0.01927812770009041.
[I 2023-10-28 20:19:37,726] Trial 5 finished with value: 1.6477125883102417 and parameters: {'lr': 1.0476654393163505e-05, 'hidden_size': 56}. Best is trial 3 with value: 0.01927812770009041.
[I 2023-10-28 20:20:00,427] Trial 6 finished with value: 0.3887619078159332 and parameters: {'lr': 7.862382718711628e-05, 'hidden_size': 167}. Best is trial 3 with value: 0.01927812770009041.
[I 2023-10-28 20:20:21,542] Trial 7 finished with value: 0.8417856097221375 and parameters: {'lr': 1.9814608668196852e-05, 'hidden_size': 131}. Best is trial 3 with value: 0.01927812770009041.
[I 2023-10-28 20:20:43,053] Trial 8 finished with value: 0.23458336293697357 and parameters: {'lr': 0.00044820307801240116, 'hidden_size': 39}. Best is trial 3 with value: 0.01927812770009041.
The best parameters are {'lr': 0.007958220298373548, 'hidden_size': 115} with a loss of 0.01927812770009041.
[I 2023-10-28 20:21:03,909] Trial 9 finished with value: 0.0469413585960865 and parameters: {'lr': 0.008093101024779201, 'hidden_size': 172}. Best is trial 3 with value: 0.01927812770009041.Process finished with exit code 0
可视化结果
import optuna
def objective(trial):
# 这里是你的模型和超参数空间
x = trial.suggest_float('x', -10, 10)
return (x - 2) ** 2
study = optuna.create_study()
study.optimize(objective, n_trials=100)
# 可视化
optuna.visualization.plot_optimization_history(study)
optuna.visualization.plot_param_importances(study)
更多推荐
所有评论(0)