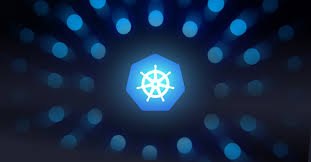
【K8S device-plugin】以 vgpu 项目分析 device-plugin、Scheduler Extender Plugin、KubeSchedulerConfiguration 关系
注意此处,若没有 KubeSchedulerConfiguration的声明配置,k8s 会认为这些(nvidia.com/gpumem、nvidia.com/gpucores)是资源设备,k8s 调度时候会进行寻找,发现所有节点上都没此资源设备配额(kubectldescribenodenodeName 可以看到每个 node 的设备资源情况),导致该 pod 调度失败。首先创建个 device
项目
- https://github.com/4paradigm/k8s-vgpu-scheduler/
k8s-vgpu-scheduler 如何实现 vgpu 的资源分配
apiVersion: v1
kind: Pod
metadata:
name: gpu-pod
spec:
containers:
- name: ubuntu-container
image: ubuntu:18.04
command: ["bash", "-c", "sleep 86400"]
resources:
limits:
nvidia.com/gpu: 2 # requesting 2 vGPUs
nvidia.com/gpumem: 3000 # Each vGPU contains 3000m device memory (Optional,Integer)
nvidia.com/gpucores: 30 # Each vGPU uses 30% of the entire GPU (Optional,Integer)
-
首先创建个 device-plugin,注册 gpu 资源(nvidia.com/gpu)
-
创建个专用于 gpu 资源的分配 kube-Scheduler 调度器(记不清名字了,此处称之为 gpu-scheduler,默认的 k8s 调度器是 default-scheduler,代码在该项目路径
pkg/scheduler
)- 此处主要利用 scheduler extender plugin机制,创建一个 http extender plugin 调度插件,用于调度 gpu pod 时(关注 nvidia.com/gpu 资源参数)
-
采用 KubeSchedulerConfiguration 声明上面http extender plugin 调度插件关注其他 gpu 资源配置参数(nvidia.com/gpumem、nvidia.com/gpucores)
-
注意此处,若没有 KubeSchedulerConfiguration 的声明配置,k8s 会认为这些(nvidia.com/gpumem、nvidia.com/gpucores)是资源设备,k8s 调度时候会进行寻找,发现所有节点上都没此资源设备配额(kubectl describe node nodeName 可以看到每个 node 的设备资源情况),导致该 pod 调度失败
-
此处逻辑和腾讯 gpu-manager 有些区别,腾讯是注册如 nvidia.com/vgpu、nvidia.com/vmem,两种设备,在 vgpu 分配时统计 vmem 情况,一起进行分配;到 vmem 分配时,就什么都不做了
-
# charts/vgpu/templates/scheduler/configmapnew.yaml # 此处是 helm chart 模板中的一个配置文件 apiVersion: v1 kind: ConfigMap metadata: name: {{ include "4pd-vgpu.scheduler" . }}-newversion labels: app.kubernetes.io/component: 4pd-scheduler {{- include "4pd-vgpu.labels" . | nindent 4 }} data: config.yaml: | apiVersion: kubescheduler.config.k8s.io/v1beta2 kind: KubeSchedulerConfiguration leaderElection: leaderElect: false profiles: - schedulerName: {{ .Values.schedulerName }} extenders: - urlPrefix: "https://127.0.0.1:443" filterVerb: filter bindVerb: bind nodeCacheCapable: true weight: 1 httpTimeout: 30s enableHTTPS: true tlsConfig: insecure: true managedResources: - name: {{ .Values.resourceName }} ignoredByScheduler: true - name: {{ .Values.resourceMem }} ignoredByScheduler: true - name: {{ .Values.resourceCores }} ignoredByScheduler: true - name: {{ .Values.resourceMemPercentage }} ignoredByScheduler: true - name: {{ .Values.resourcePriority }} ignoredByScheduler: true - name: {{ .Values.mluResourceName }} ignoredByScheduler: true - name: {{ .Values.mluResourceMem }} ignoredByScheduler: true
-
-
由于现在新增一个 gpu-scheduler,同时还有默认的 k8s default-scheduler,那么如何选择设置呢?—— k8s mutate webhook 机制
-
mutate webhook 会在 pod 到 scheduler 前生效,该 mutate webhook 作用主要就是,查看 pod 是否有配置
nvidia.com/gpu
资源需求,若有的话,就会更改 pod.spec 中 schedulerName,设置为 gpu-scheduler(因为不更改的话,会默认配置 default-scheduler,此 scheduler 无 gpu 调度逻辑) -
# charts/vgpu/templates/scheduler/webhook.yaml # 此处是 helm chart 模板中的一个配置文件 apiVersion: admissionregistration.k8s.io/v1 kind: MutatingWebhookConfiguration metadata: name: {{ include "4pd-vgpu.scheduler.webhook" . }} webhooks: - admissionReviewVersions: - v1beta1 clientConfig: {{- if .Values.scheduler.customWebhook.enabled }} url: https://{{ .Values.scheduler.customWebhook.host}}:{{.Values.scheduler.customWebhook.port}}{{.Values.scheduler.customWebhook.path}} {{- else }} service: name: {{ include "4pd-vgpu.scheduler" . }} namespace: {{ .Release.Namespace }} path: /webhook port: {{ .Values.scheduler.service.httpPort }} {{- end }} failurePolicy: Fail matchPolicy: Equivalent name: vgpu.4pd.io namespaceSelector: matchExpressions: - key: 4pd.io/webhook operator: NotIn values: - ignore objectSelector: matchExpressions: - key: 4pd.io/webhook operator: NotIn values: - ignore reinvocationPolicy: Never rules: - apiGroups: - "" apiVersions: - v1 operations: - CREATE resources: - pods scope: '*' sideEffects: None timeoutSeconds: 10
-
逻辑图可视化理解
1 | Device-Plugin 上报逻辑
-
部署后,kubelet 会调用 Device Plugin 的 Register 方法,将该 Device Plugin 负责的资源设备名 ResourceName(nvidia.com/gpu) 注册到 kubelet 中
-
// pkg/device-plugin/nvidiadevice/nvinternal/plugin/server.go // Register registers the device plugin for the given resourceName with Kubelet. func (plugin *NvidiaDevicePlugin) Register() error { conn, err := plugin.dial(pluginapi.KubeletSocket, 5*time.Second) if err != nil { return err } defer conn.Close() client := pluginapi.NewRegistrationClient(conn) reqt := &pluginapi.RegisterRequest{ Version: pluginapi.Version, // dfy: 与资源管理 server 端通信的 socket Endpoint: path.Base(plugin.socket), // dfy: 注册资源名称 ResourceName: string(plugin.rm.Resource()), Options: &pluginapi.DevicePluginOptions{ GetPreferredAllocationAvailable: true, }, } _, err = client.Register(context.Background(), reqt) if err != nil { return err } return nil }
-
-
Kubelet 调用 Device Plugin 的 ListAndWatch 方法,监听负责资源设备的变化,并同步到 Node 中(可以通过 kubectl describe node xx 查看该设备资源)
-
// pkg/device-plugin/nvidiadevice/nvinternal/plugin/server.go // ListAndWatch lists devices and update that list according to the health status func (plugin *NvidiaDevicePlugin) ListAndWatch(e *pluginapi.Empty, s pluginapi.DevicePlugin_ListAndWatchServer) error { s.Send(&pluginapi.ListAndWatchResponse{Devices: plugin.apiDevices()}) for { select { case <-plugin.stop: return nil case d := <-plugin.health: // FIXME: there is no way to recover from the Unhealthy state. d.Health = pluginapi.Unhealthy klog.Infof("'%s' device marked unhealthy: %s", plugin.rm.Resource(), d.ID) s.Send(&pluginapi.ListAndWatchResponse{Devices: plugin.apiDevices()}) } } }
-
2 | Device-Plugin 分配资源逻辑
- 可以把 kubelet 看做客户端, Device Plugin 看做为 Server 端
- 他们之间的通信,通过放置在
/var/lib/kubelet/device-plugins
目录下的 socket 来进行通信 - kublet 会调用 Device Plugin 的 Allocate 函数,根据 pod 的 request 情况来申请需要的资源设备信息
3 | Scheduler Extender Plugin
- Pod 中 request 可能有多种资源设备(如 nvidia.com/gpu、nvidia.com/gpumem、nvidia.com/gpupercentage 等),但是目前只注册了一种资源设备(就是 nvidia.com/gpu,也就对应上面的 gpu.sock 文件)
- 其他两种没注册(nvidia.com/gpumem、nvidia.com/gpupercentage),若在 pod request 中填写,便会在 Pod 调度时,无法识别这两种资源,导致 Pending 状态
- 当目前我们想要这三种资源设备都交由同一个 Nvidia Device Plugin 处理,这个该怎么做呢?
- 可以填写 Scheduler 的 KubeSchedulerConfiguration 文件,指定一个 Scheduler Extender Plugin 关注这三种资源,从而为该 Pod 选择一个合适的 Node
更多推荐
所有评论(0)