RNN时间序列预测(2)-Tensorflow入门,RNN操作
tensorflow
一个面向所有人的开源机器学习框架
项目地址:https://gitcode.com/gh_mirrors/te/tensorflow

·
Title | Content |
---|---|
原文 | https://www.tensorflow.org/tutorials/recurrent |
说明 | 因为要做RNN的时间序列分析,需要用到LSTM模型,以下是学习摘要 |
模型LSTM
模型包含一个LSTM cell,在一个时刻处理一个输入,并计算下一个可能出现值的概率。模型初始化为0向量,处理完输入后更新。为了计算效率,设定最小批处理数为batch_size
batch_size:最小批处理数,但是每一批的对应index上的值,应该是同一时刻的
lstm_size:隐藏层的feature数目,也就是node个数,用户设定
lstm = tf.controb.rnn.BasicLstmCell(lstm_size)
//其实就是类似初始化一个batch_size*lstm_size的矩阵, 不太确定lstm.state_size是否等于lstm_size???
state = tf.zeros([batch_size, lstm.state_size])
probabilities = []
loss = 0.0
for current_batch in dataset:
//处理完每一批数据后,state更新
output, state = lstm(current_batch, state)
//LSTM输出可用于下一个数据的预测
logits = tf.matmul(output, softmax_w)+softmax_b //上一篇提到过的概率计算方式
probabilities.append(tf.nn.softmax(logits))
loss += loss_function(probabilities, target)
截断反向传播
用于RNN设计中,其输出决定入任意距离的输入,为了解决法相传播的效率问题,创建一个“展开”的网络。包含固定个数num_steps的LSTM输入和输出,每一步其实就是一个类似RNN的过程。做法是,每次喂给网络num_steps个输入,然后它会回传。
我的理解就是,给了num_steps个RNN,每一个代表一个时刻,对应时刻的值输入到对应的网络,同时当前时刻的网络会接受来自前一个网络的输入或输出。
tensorflow
一个面向所有人的开源机器学习框架
项目地址:https://gitcode.com/gh_mirrors/te/tensorflow
step的迭代处理:
//每次迭代,输入数据的占位符
words = tf.placeholder(tf.int32, [batch_size, num_steps]) //每次喂给网络num_steps个输入值,一批次处理batch_size次
lstm = tf/conrib.rnn.BasicLSTMCell(lstm_size)
state = tf.zeros([batch_size, lstm.state_size])
//对于每一个step(其实就是每一个时刻),通过batch_size个当前时刻的值,来训练时刻的网络
for i in range(num_steps):
output, state = lstm(current_batch, state)
...
final_state = state
全局迭代处理:
numpy_state = initial_state.eval() //每次batch处理完成后,存储LSTM的state
total_loss = 0.0
for current_batch in dataset:
numpy_state, current_loss = session.run([final_state, loss], feed_dict={initial_state:numpy_state, words:current_batch})
total_loss += current_loss
LSTM多层叠加
为了增加模型的表诉能力,可以添加多层LSTM,将第一层的输出作为第二个的输入
lstm = tf.contrib.rnn.BasicLSTMCell(lstm_size, state_is_tuple=false)
stacked_lstm = tf.contrib.rnn.MultiRNNCell([lstm]*num_of_layers, state_is_tupe=false)
initial_state = state = stacked_lstm.zero_state(batch_size, tf.floatt32)
for i in range(num_steps):
output, state = stacked_lstm(words[:,i], state)
...
final_state = state
阅读全文
AI总结
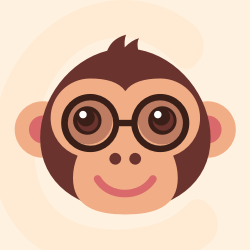



一个面向所有人的开源机器学习框架
最近提交(Master分支:2 个月前 )
4f64a3d5
Instead, check for this case in `ResolveUsers` and `ResolveOperand`, by querying whether the `fused_expression_root` is part of the `HloFusionAdaptor`.
This prevents us from stepping into nested fusions.
PiperOrigin-RevId: 724311958
2 个月前
aa7e952e
Fix a bug in handling negative strides, and add a test case that exposes it.
We can have negative strides that are not just -1, e.g. with a combining
reshape.
PiperOrigin-RevId: 724293790
2 个月前
更多推荐
相关推荐
查看更多
tensorflow

一个面向所有人的开源机器学习框架
tensorflow

TensorFlow for R
TensorFlow

Project containig related material for my TensorFlow articles
目录
所有评论(0)